Trusting Big Data requires understanding its Data Lineage. Without Data Lineage, Big Data becomes synonymous with the last phrase in a game of telephone.
Michelle is right - you have to know the system of record and the data flow for the data that you are using, and you need to know that for ALL data that you use. You need to also understand the quality of that data and what to do when values are missing (preferably that should never happen but we all live in the real world with legacy systems).
This is a blog containing data related news and information that I find interesting or relevant. Links are given to original sites containing source information for which I can take no responsibility. Any opinion expressed is my own.
Sunday, 30 April 2017
Saturday, 29 April 2017
Google is using AI to help humans and computers communicate through art by Rob Verger via @PopSci
Google went big on art this week. The company launched a platform to help people who are terrible at art communicate visually. It also published research about teaching art to another terrible stick-figure drawer: a neural network.
A strange use but clever anyway.
A strange use but clever anyway.
Friday, 28 April 2017
WEBINAR: Less artificial, more intelligence – The future of analytics - 4 May 2017
Web Seminar Less artificial, more intelligence – The future of analytics
May 04, 2017 | 2 PM ET/11 AM PT
Hosted by Information Management
Hosted by Information Management
Artificial Intelligence (AI) is everywhere these days, but most businesses have not cracked the code on how to use AI to drive competitive advantage or leverage their Big Data. AI is now less ‘artificial’, making it practical to weave these sophisticated technologies into business processes and everyday work life. However, there is still a need for AI to be more 'intelligent' – helping organisations achieve the previously impossible, whether it’s in fraud detection or manufacturing automation.
Join this webinar and learn more about:
- How organisations can extract the ‘intelligence’ in AI
- The common pitfalls in trying to exploit AI
- What is needed to fully leverage AI to drive competitive advantage
- The new analytic techniques and innovations currently being used by forward-looking organisations today
Featured Presenters:
Moderator: Jim Ericson Consultant, Editor Emeritus Information Management | Speaker: Scott Zoldi Chief Analytics Officer FICO |
- Register here
Infrastructure, scaling and staffing top barriers to analytics success by David Weldon via @infomgmt
While the number of firms implementing big data projects is on the rise, many cite a number of significant challenges.
A good list of barriers. I would say for staffing it's actually only for certain skills or disciplines as there are still shortages for those. The solution is not quick and needs a concentrated effort in training and education. However that is not the complete solution as experience is also needed.
A good list of barriers. I would say for staffing it's actually only for certain skills or disciplines as there are still shortages for those. The solution is not quick and needs a concentrated effort in training and education. However that is not the complete solution as experience is also needed.
Thursday, 27 April 2017
How to Be a Data Scientist: Data Science Skill Development by Paramita Ghosh via @Dataversity
A great article discussing the skills needed to be a Data Scientist and how to develop them.
Very clear and detailed article that needs to be read carefully and absorbed.
Very clear and detailed article that needs to be read carefully and absorbed.
Wednesday, 26 April 2017
SLIDESHOW: 8 top platforms for master data governance by David Weldon via @infomgmt
Informatica Data Director, IBM Stewardship Center and Collibra Data Governance Center are among the top MDG picks from The MDM Institute.
These look good and we have no excuse for not dong this properly if we have a tool like these to help. I only have direct experience of IDD which seemed ok.
These look good and we have no excuse for not dong this properly if we have a tool like these to help. I only have direct experience of IDD which seemed ok.
Tuesday, 25 April 2017
Becoming a Data Scientist: Profiling Cisco’s Data Science Certification Program by Megan Risdal via @kaggle
Great blog by Megan Risdal - At Cisco, today's subject matter experts are tomorrow's data scientists. Learn how Cisco scales skills and knowledge across their organisation.
The level 0 courses are public courses so you can definitely start with those.
The level 0 courses are public courses so you can definitely start with those.
Monday, 24 April 2017
Basics of Entity Resolution with Python and Dedupe by Kyle Rossetti and Rebecca Bilbro via @DistrictDataLab
Great blog by Kyle Rossetti and Rebecca Bilbro explains how to disambiguate records that correspond to real-world entities across and within datasets using the Python dedupe package.
Contains code and examples so you can really understand it and easily replicate in your own work.
Contains code and examples so you can really understand it and easily replicate in your own work.
Sunday, 23 April 2017
So You Want to Be a Data Scientist? – It’s Complicated by @eliza_medley via @Datafloq
Data Science is the sexiest tech career field - in this article Eliza Medley contains a lot of great advice on the best paths to move towards a career in Data Science.
Kaggle is a great site and there is a test for membership so don't assume it is easy to join. I actually wrote some R code to work out the answers.
Kaggle is a great site and there is a test for membership so don't assume it is easy to join. I actually wrote some R code to work out the answers.
Saturday, 22 April 2017
5 Data Management Mistakes to Avoid during Data Integration Projects by @mairabay via @hlsdk
In this article Canada based Maira Bay de Souza of Product Data Lake Technologies shares her view on data integration and the mistakes to avoid doing that.
I agree with her observations but feel that there needs to be more focus on the data that this article describes (although that could be because I spent so many years doing the detailed design for data integrations and loads on a data warehouse).
My thoughts are:
You need detailed documentation of the data at source, target and any processing in between. That documentation should cover formats, values, lookups, defaults, translations, timezones, currencies, master data location/values and anything else you can find.
You need to think about if you need to handle Slowly Changing Dimensions at all stages of the integration as they could impact your interface (I don't think they are something that only affects a Data Warehouse)
I agree with her observations but feel that there needs to be more focus on the data that this article describes (although that could be because I spent so many years doing the detailed design for data integrations and loads on a data warehouse).
My thoughts are:
You need detailed documentation of the data at source, target and any processing in between. That documentation should cover formats, values, lookups, defaults, translations, timezones, currencies, master data location/values and anything else you can find.
You need to think about if you need to handle Slowly Changing Dimensions at all stages of the integration as they could impact your interface (I don't think they are something that only affects a Data Warehouse)
Friday, 21 April 2017
WEBINAR: IoT: Tackling the Data Management and Analytics Challenge - 27 April 2017

Overview
Title: IoT: Tackling the Data Management and Analytics Challenge
Date: Thursday, April 27, 2017
Time: 09:00 AM Pacific Daylight Time
Duration: 1 hour
Summary
IoT: Tackling the Data Management and Analytics Challenge
Data management and analytics tools will be the core enabler of new values created by the intersection of the Internet of Things (IoT), people and the physical world. However, challenges related to IoT data management and analytics are currently hindering the implementation of increasingly complex IoT applications that will drive this value.
By providing an offering that addresses these key IoT data challenges, the Vertica Analytics Platform goes far beyond fragmented and legacy systems that are simply not equipped to handle the challenges of IoT data. Harbor Research recently authored a white paper on the HPE Vertica Analytics Platform, which details how Vertica addresses the myriad challenges posed by IoT data.
Join us for this latest IoT Central webinar and you will learn:
- What is really meant by IoT data management and analytics
- Why legacy solutions are not suited to handle IoT data
- How Vertica’s key functionalities and ecosystem address the challenges of IoT data
- How equipment manufacturers and IoT platform providers can act as catalysts for delivering IoT data management and analytics solutions
Speakers:
Alex Glaser, Director of Development - Harbor Research
Walter Scheib, Senior Associate - Harbor Research
Alex Glaser, Director of Development - Harbor Research
Walter Scheib, Senior Associate - Harbor Research
Hosted by:
David Oro, Editorial Director -- IoT Central
Register here

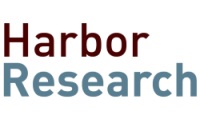
Firing on All Cylinders: The 2017 Big Data Landscape by/via @mattturck
Great overview of the Big Data ecosystem by Matt Turck of FirstMark Capital. Includes a discussion of what's hot now, where things are going, and a comprehensive map of important players.
This is a great post and the chart is great even if it is a little out of date.
This is a great post and the chart is great even if it is a little out of date.
Thursday, 20 April 2017
WEBINAR: Exploiting data lakes - 27 April 2017
Web Seminar Exploiting data lakes
Apr. 27, 2017 | 2 PM ET/11 AM PT
Hosted by Information Management
Hosted by Information Management
The great promise of a data lake is: all data housed in one place, for all that want it, when they want it. While that may sound attractive at a high level, too frequently the results look more like a data swamp, where users can’t address data real-time or they can’t trust the data.
Among the topics to be discussed:
- What are the advantages of a data lake approach for housing an organisation's data?
- What are the greatest challenges or concerns with a data lake environment?
- What steps must an organisation take to ensure that data in a data lake is trustworthy and accessible?
Featured Presenters:
Register here
Detecting fraud through data analysis by Richard Fowler via @infomgmt
It seems that these schemes often start as an honest mistake, and if no one notices, then a fraudster 'accidentally' does it again.
It is critical to monitor transactions in order to pick up fraud as well as closing the loophole that was exploited in order to create it. In a perfect world it would be great if systems were designed, coded and tested perfectly but holes can and will be found so monitoring and closure is key to minimise losses.
It is critical to monitor transactions in order to pick up fraud as well as closing the loophole that was exploited in order to create it. In a perfect world it would be great if systems were designed, coded and tested perfectly but holes can and will be found so monitoring and closure is key to minimise losses.
Wednesday, 19 April 2017
WEBINAR: Graph-based MDM: Why relational DBMS aren't relational enough - 25 April 2017
Web Seminar Graph-based MDM: Why relational DBMS aren't relational enough
Apr. 25, 2017 | 2 PM ET/11 AM PT
Hosted by Information Management
Hosted by Information Management
Success increasingly depends on your ability to discern customer needs, preferences, and buying behaviors through data that’s ever more diverse and voluminous. MDM is supposed to help you with this digital transformation. But conventional MDM may not deliver the full contextual insight your business stakeholders need.
Attend this eye-opening webinar to learn how graph/NoSQL databases dramatically enhance MDM—empowering you to out-analyze, out-decide, and out-innovate the competition.
Our expert MDM thought-leaders will show you:
- How graph/NoSQL models uncover complex, subtle relationships between disparate data
- Why less MDM structure can yield major advantages in analytics and decision-making
- How graph technology can reduce your need for high-cost data science skills
Featured Presenters:
Moderator:
Lenny Leibmann Contributing Editor SourceMedia |
Sponsored By:
Register here
Alternative Data and Machine Learning by @noelbambrick via @_aylien
The landscape of data is ever-changing, meaning analysts need to evolve both their thinking and data collection methods to stay ahead of the curve. In many cases, data that might have been considered unique, uncommon or unattainably expensive just a few years ago is now widely used and often very affordable.
I love this blog from Aylien. It gives great examples of Alternative Data and the way it can be used. No one can read this without understanding and grasping the possibilities.
I love this blog from Aylien. It gives great examples of Alternative Data and the way it can be used. No one can read this without understanding and grasping the possibilities.
Tuesday, 18 April 2017
The subtleties of data sovereignty by Andrew White via @infomgmt
Determining sovereignty includes where the data was formed, where it is stored, and where an inquiry is being undertaken from, says Andrew White.
This is an absolute minefield. Having worked for a global company which has it's headquarters and data centre in the US, but working in an office in the UK I can understand some of the subtleties of the various rules and regulations. It's not even just about the sovereignty - it's also other areas around it too like different legal requirements for data retention and the different levels of privacy for different countries/regions too.
This is an absolute minefield. Having worked for a global company which has it's headquarters and data centre in the US, but working in an office in the UK I can understand some of the subtleties of the various rules and regulations. It's not even just about the sovereignty - it's also other areas around it too like different legal requirements for data retention and the different levels of privacy for different countries/regions too.
Monday, 17 April 2017
How GoDaddy powers its team with big data analytics by Bob Violino via @infomgmt
Web services company creates a self-service environment with Tableau as its primary visualization tool.
Having used Excel, Tableau and SQL to do that kind of thing I can completely understand the benefits of using a tool like Tableau - I'm sure they are making much better decisions by using their data in a much better way which has probably paid for the cost of Tableau many times over.
Having used Excel, Tableau and SQL to do that kind of thing I can completely understand the benefits of using a tool like Tableau - I'm sure they are making much better decisions by using their data in a much better way which has probably paid for the cost of Tableau many times over.
Sunday, 16 April 2017
How to stay out of analytic rabbit holes: avoiding iloops and their traps by Karolis Urbonas via @Cyborguscom
"'What if we add these variables? is a deadly type of a question that can ruin your analytic project. Now, while curiosity is the best friend of a data scientist, there’s a curse that comes with it—some call it analysis paralysis, others overanalysis, but I call these situations analytic rabbit holes." Here's how to avoid investigation loops and their traps.
We can all do with this great advice - all I can say is save each version as you go so you can easily go back to a version that works great.
We can all do with this great advice - all I can say is save each version as you go so you can easily go back to a version that works great.
Saturday, 15 April 2017
What’s the Difference Between Artificial Intelligence, Machine Learning, and Deep Learning? by Nvidia via @odsc
Handy guide as it's not always easy to tell the difference between them.
Friday, 14 April 2017
5 Reasons Why Startups Must Use Business Intelligence Tools By Lewis Robinson via IT Business Net
Many small business startups tend to shy away from business intelligence tools (BI) that enable them to manage and analyse data. Primarily because they are very expensive, difficult to use and are typically reserved for large corporations that have the wherewithal to support its complexities. Although larger corporations have the resources to hire dedicated teams and staff members that are needed to manage software as well as interpret and report data, business intelligence tools can also benefit new startup businesses as well.
I agree with him - everyone can get some kind of insight and there are free tools that are adequate to get a small fledgling business going.
I agree with him - everyone can get some kind of insight and there are free tools that are adequate to get a small fledgling business going.
Thursday, 13 April 2017
Data to see tenfold increase worldwide by 2025 by David Weldon via @infomgmt
Virtually every organisation will be affected, putting new pressure on creation, collection, utilisation and management.
Yes I can only start to think about all the ways that data quantity is going to increase, however without associated QUALITY the data is not necessarily of any use or may even point you in the wrong way.
Yes I can only start to think about all the ways that data quantity is going to increase, however without associated QUALITY the data is not necessarily of any use or may even point you in the wrong way.
Wednesday, 12 April 2017
Python Deep Learning Frameworks Reviewed by Madison May via @indicoData
Here's a short overview of some of the leading Python deep learning frameworks, including Theano, Lasagne, Blocks, TensorFlow, Keras, MXNet, and PyTorch.
This is a great blog post and well worth reading/bookmarking.
This is a great blog post and well worth reading/bookmarking.
Tuesday, 11 April 2017
Why Big Data Is Not Truth by Quentin Hardy via @nytimesbits
The word “data” connotes fixed numbers inside hard grids of information, and as a result, it is easily mistaken for fact.
This is a great article and well worth reading.
This is a great article and well worth reading.
Monday, 10 April 2017
7 forces driving modern business intelligence growth by David Weldon via @infomgmt
More focus is being placed on business-led, agile analytics and self-service features rather than IT-led system-of-record reporting.
I completely agree - I've definitely observed that kind of transformation myself.
I completely agree - I've definitely observed that kind of transformation myself.
Sunday, 9 April 2017
4 ways to think like a data scientist (to become one) by Karolis Urbonas via @Cyborguscom
Karolis Urbonas, head of business intelligence and data science of Amazon Devices, wanted to live in the middle cross-section of the famous data science Venn diagram (that's right, the "unicorn data scientist" cross-section). In this post, he explains the "four rules that have helped him survive in the data science world."
This is a great set of rules to follow. Read this and print it out so you cannot forget it.
This is a great set of rules to follow. Read this and print it out so you cannot forget it.
Saturday, 8 April 2017
SLIDESHOW: Leading AI companies in healthcare via @infomgmt
Friday, 7 April 2017
Historians versus futurists – the battle of analysts? by David Cokins via @infomgmt
Some analysts dig deep into historical information to glean insights once hidden. Others are obsessed with predictive analytics and Big Data to foresee the future.
I really like this opinion piece and think it is worth thinking about the two faces of analysis and what you are currently doing.
I really like this opinion piece and think it is worth thinking about the two faces of analysis and what you are currently doing.
Thursday, 6 April 2017
Firms have no time for anything less than real-time data by David Weldon via @infomgmt
The typical organisation today is drowning in data, and IT and business leaders need immediate insights to drive innovation, says Zoomdata executive.
I agree and you can get some really great insights based on real-time data.
I agree and you can get some really great insights based on real-time data.
Wednesday, 5 April 2017
Learning not to ‘over think’ predictive analytics by David Weldon via @infomgmt
Most firms are more ready than they realise to implement the technology, and they fail to see the countless opportunities at hand.
I agree with the comment at the end of the article - tools have to be built for everyone not just the data science community.
I agree with the comment at the end of the article - tools have to be built for everyone not just the data science community.
Tuesday, 4 April 2017
WEBINAR: When Your Big Data Seems Too Small: Accurate Inferences Beyond the Empirical Distribution - 9 March 2017
When Your Big Data Seems Too Small: Accurate Inferences Beyond the Empirical Distribution
March 09, 2017 | 10-11 am PT
A recording of this webinar will be made available within a week of the live session.
Many of the techniques and algorithms that are used in machine learning and data sciences assume that the empirical distribution of the available data is an accurate approximation of the primary phenomena being investigated. However, when dealing with complex or high dimensional distributions, even large datasets can fail to accurately represent its core. As examples, in large genomic datasets many rare genetic variants are unobserved, and in a large natural language corpus, many reasonable sequences of five words might not be observed.
Join Stanford’s Dr. Gregory Valiant as he discusses the difficulties of and solutions for making accurate inferences in this challenging regime, in which the empirical distribution of the available data is misleading. Learn how to extract accurate information about the underlying distribution, including information about the portion that has not been observed in the given dataset.
You will learn:
- An intuitive approach for reasoning about the distribution that underlies a given dataset
- Techniques that leverage this intuition, and reveal the structure of the underlying distribution---including the structure of the unseen portion of it from which no datapoints have been observed
- Practical implications of these techniques for the analysis of genomic datasets, including how to estimate the value of sequencing additional human genomes
About the Speaker
Presented By
Stanford's Databases and the Foundations in Computer Science graduate certificate programs
Register here
WEBINAR: Data Science Made Simple with SPSS - 7 March 2017

Overview
Title: Data Science Made Simple with SPSS
Date: Tuesday, March 07, 2017
Time: 09:00 AM Pacific Standard Time
Duration: 1 hour
Summary
Data Science Made Simple with SPSS
For decades, IBM SPSS® Statistics has been the trusted data analytics package for statisticians, researchers, and business analysts. That’s because it offers superior capabilities, flexibility and usability that are not available in traditional statistical software. Now, IBM SPSS Statistics is available by subscription, offering even greater speed and ease of use than ever before—with no more software licenses or worrying about version updates.
Join us for an overview of the new IBM SPSS Statistics Subscription. In this Data Science Central webinar, learn how you can start enjoying the benefits of a powerful, affordable data analysis tool that can help you more easily:
Join us for an overview of the new IBM SPSS Statistics Subscription. In this Data Science Central webinar, learn how you can start enjoying the benefits of a powerful, affordable data analysis tool that can help you more easily:
- Access, manage, and analyse virtually any kind of data set
- Gain reliable results with a broad range of tests and procedures
- Use R and Python to further extend your capabilities
Whether you are a beginner or an experienced analyst or statistician, IBM SPSS Statistics Subscription software puts the power of advanced statistical analysis at your fingertips. Register for this Data Science Central webinar to learn how you can start getting faster, more accurate results from your data today.
Speaker: Douglas Stauber, Offering Manager - IBM SPSS Statistics
Hosted by: Bill Vorhies, Editorial Director -- Data Science Central
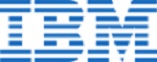
Register here
Labels:
DATA,
DATA SCIENCE,
PYTHON,
R,
SPSS,
STATISTICS
Ideas on interpreting machine learning by Patrick HallWen PhanSriSatish Ambati via @h2oai @oreillymedia
Mix-and-match approaches for visualising data and interpreting machine learning models and results.
This is great and very comprehensive.
This is great and very comprehensive.
Monday, 3 April 2017
SLIDESHOW: The 14 leading products for predictive analytics and machine learning by David Weldon via @infomgmt
IBM SPSS Modeler SAS Analytics Suite and KNIME Analytics Platform are among the best bets in Forrester’s Wave Report.
Interesting list over 19 slides.
Interesting list over 19 slides.
Sunday, 2 April 2017
50 Companies Leading The AI Revolution, Detailed by Thuy T. Pham via @kdnuggets
Here she details 50 companies leading the Artificial Intelligence revolution in AD Sales, CRM, Autotech, Business Intelligence and analytics, Commerce, Conversational AI/Bots, Core AI, Cyber-Security, Fintech, Healthcare, IoT, Vision, and other areas.
This is a great list for Thuy.
This is a great list for Thuy.
Saturday, 1 April 2017
Distill: Supporting Clarity in Machine Learning by Shan Carter and Chris Olah via @googleresearch
A joint launch between OpenAI, Google Brain, and YCombinator, Distill aims to provide a better mechanism for disseminating research on ML.
This looks quite interesting.
This looks quite interesting.
Subscribe to:
Posts (Atom)